Research Interests
My research interests are in the areas of Machine Learning, Data Science and Mining, and Artificial Intelligence in general. More specifically, my interests are a bit eclectic, but there are two major themes:
-
Multi-label classification, multi-target and structured-output prediction (modelling multiple interconnected tasks or subtasks)
-
Learning from sequential data and data streams, which includes sequential decision making, autonomous agents, and reinforcement learning
The two themes are often connected; since sequential implies multiple and often vice versa. With regard to both I am specifically interested in themes of explainability, uncertainty analysis, robustness and reliability, domain shift/concept drift, transfer learning and continual learning.
I’ll use any tools suited to the task, including deep neural network architectures and deep learning, probabilistic graphical models, Monte Carlo methods and MCMC and other methods from computational statistics, and classical machine learning algorithms such as decision trees and random forests.
I also enjoy tackling real-world problems (some listed below), and data science applications; including sensor networks and sensory data, transport and energy systems and medicine, biology and the natural sciences.
See a short presentation (pdf) of some topics of interest and of the wider DaSciM team (see also the DaSciM team web page). As follows a selection of research activity, a lot of which involves the work of PhD students (and other colleagues).
Topic: Multi-label Classification and Multi-Target Prediction
In multi-label classification, multiple target variables are associated with each instance, as opposed to the traditional supervised learning problem where a single class label is assigned to each instance. This involves standard tasks such as image and text categorization, as well as recommender systems, missing value imputation, and more general structured-output prediction problems such as time series and trajectory forecasting.
Particular challenges: explainability and interpretability, distribution learning, distribution shift.
Methods of choice: classifier chains, regressor chains, probabilistic graphical models.
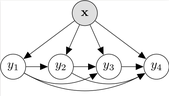
Highlights:
-
[Jul 2023] On the Connection between multi-label learning and cross-domain transfer learning.
-
[May 2022] When, under uncertainty, the predictive posterior distribution can be multi-modal: Multi-output regression for modal estimates.
-
[Jan 2021] Our followup review paper Classifier Chains: A Review and Perspectives providing a review of 10 years of classifier chains (and here, a set of related slides).
-
[May 2020] In our paper Probabilistic Regressor Chains with Monte Carlo Methods we look at how the chaining mechanism can apply to continuous output spaces.
-
[Sep 2019] Our paper on Classifier Chains for Multi-label Classification wins the Test of Time Award at ECML-PKDD 2019 [Slides].
-
[2016] We looked at the connections of classifier chains to deep learning.
-
[2019] And on the choice of metric in multi-label classification.
-
[2016] The MEKA framework for multi-label learning and evaluation is published in JMLR MLOSS. See multi-label software.
-
[2014] We won the 2014 LSHTC4 Kaggle competition on Large Scale Hierarchical Text Classification, using an approach based on meta labels.
Topic: Learning from Data Streams
Many real-world applications are found in the context of data streams, where data instances arrive rapidly and continuously in a theoretically-infinite stream, for example from sensors, online social media and text streams. Reinforcement learning is typically carried out in such a scenario; where a stream of observations comes from an environment.
Challenges: learning with weak, partial, noisy, and delayed labels; adaptation to concept drift; online learning.
Applications: anomaly and event detection, time series and trajectory forecasting, complex energy systems.
Highlights:
-
[Jan 2023] We provide an update on data stream learning, in particular a fresh look at applications
-
[2020 — 2021] We challenge assumptions made in the data streams literature, arguing that many don’t need to be met it practice. We also show that concept drift implies temporal dependence and should be evaluated as such.
-
[-- 2023] Supervision (true labels) may be delayed or are often never available (hence, semi-spervised/partially-labeled streams)
-
[2018 — 2020] We show that Hoeffding trees are not actually instance-incremental models, and in fact theoretically they can be seen as stable learners, precisely unsuited for dynamic data streams; so introduce streaming random patches.
-
[2014 — 2018] Batch-incremental approximations often work well (no need for online learning), or can be unified.
Software: software for streams.
Application: Modelling and diagnosis of sleep disorders (Updated: 04/2019)
See slides, e.g., Machine Learning and AI in Healthcare.
Working with Olivier Pallanca (neurophysiologist) we are building predictive models for diagnosing different types of insomnia and, more importantly, predicting the response to different treatment options based on the personal characteristics of each patient ; with data such as psychological questionnaires, overnight EEG and ECG signals, skin conductance, eye movement, reaction time. This involves a number of subtasks such as event detection in sequences and streams involving multiple correlated outputs. Interpretability of models is a key aspect.
Application: Trajectory prediction (2015)
Given only a week or so of location data from a mobile phone device, it was possible to make reasonably accurate predictions about the a traveller’s route and future destination in an urban setting. Here is a Demo Animation (the captions explain what is going on)
— using real data collecting in the greater Helsinki area.
Application: Modelling tree growth in Scots pine (2015)
We worked with forestry scientists from the University of Helsikni to model intra-annual growth of Scots pine trees at sites in Finland and France using time series and machine learning models (paper).
Application: Tracking on very low-power sensor motes (2012)
We formulated and implementing a distributed particle filter on very low-power motes (4 MHz CPU) for real-time target tracking. This video of a demo of testbed deployment shows tracking using only light sensor observations.